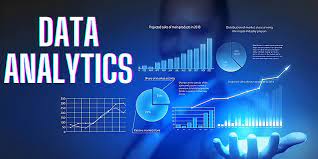
1. What is Data Analytics?
Using Data Analytics to Drive Business Decisions
Introduction: The Role of Data Analytics in Modern Business
In today’s fast-paced business environment, making informed decisions is critical to staying competitive. Data analytics provides organizations with the tools and insights needed to understand market trends, customer behaviors, and operational efficiencies. By leveraging analytics, businesses can transform raw data into actionable insights, enabling smarter decisions that drive growth and innovation.
This article explores how data analytics impacts decision-making, the types of analytics available, and practical ways to implement data-driven strategies.
1. What is Data Analytics?
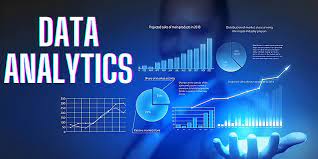
Data analytics refers to the process of examining raw data to uncover patterns, trends, and insights that inform decision-making. It involves collecting, cleaning, analyzing, and interpreting data using various tools and methodologies. Businesses use data analytics to:
- Identify market trends and opportunities.
- Improve operational efficiency.
- Enhance customer experience.
- Optimize marketing campaigns.
- Forecast future performance.
Key Types of Data Analytics:
- Descriptive Analytics: Explains what happened (e.g., sales reports, website traffic analysis).
- Diagnostic Analytics: Analyzes why something happened (e.g., identifying the root causes of customer churn).
- Predictive Analytics: Forecasts future trends based on historical data (e.g., sales forecasting, demand prediction).
- Prescriptive Analytics: Recommends actions based on analytics outcomes (e.g., resource allocation, marketing optimization).
2. Why Data Analytics is Essential for Decision-Making
a. Data-Driven Insights
Analytics provides evidence-based insights that remove guesswork from decision-making. For example, a retail business can analyze customer purchasing patterns to identify best-selling products and adjust inventory accordingly.
b. Improved Efficiency
Data analytics streamlines operations by identifying bottlenecks and inefficiencies. For example, manufacturers can use analytics to optimize supply chain processes, reducing waste and costs.
c. Enhanced Customer Understanding
By analyzing customer data, businesses can gain deeper insights into preferences, behaviors, and pain points, allowing them to deliver personalized experiences that build loyalty.
d. Risk Management
Predictive analytics helps businesses anticipate risks and take proactive measures. For example, financial institutions use analytics to detect fraud and assess credit risks.
Stat Insight: A study by McKinsey found that data-driven organizations are 23 times more likely to acquire customers, 6 times more likely to retain customers, and 19 times more likely to be profitable.
3. How to Use Data Analytics to Drive Business Decisions
a. Define Clear Goals
Identify specific business questions or challenges you want to address with data analytics. For example:
- “What factors influence customer retention?”
- “Which marketing channels yield the highest ROI?”
b. Collect and Organize Data
Data comes from various sources, including:
- Internal Sources: Sales reports, CRM systems, financial data.
- External Sources: Market research, social media, industry reports.
- IoT Devices: Sensors, GPS, and other connected devices.
Tip: Use data management tools to organize and integrate data from different sources for a comprehensive view.
c. Use the Right Tools and Techniques
Leverage analytics tools that align with your business needs. Popular tools include:
- Google Analytics: For website performance and user behavior insights.
- Tableau and Power BI: For data visualization and business intelligence.
- R and Python: For advanced statistical analysis and machine learning.
Analytical Techniques:
- Regression analysis for identifying relationships between variables.
- Cluster analysis for segmenting customers or products.
- Sentiment analysis for understanding customer feedback.
d. Analyze and Interpret Data
Focus on actionable insights rather than overwhelming yourself with data. For example:
- A restaurant chain might analyze foot traffic data to optimize opening hours.
- An e-commerce store can use customer segmentation to tailor marketing campaigns.
e. Implement and Monitor Changes
Use insights from analytics to make data-driven decisions, and track their impact over time. Adjust strategies as needed based on performance metrics.
Example: A company notices through analytics that its email campaigns have low engagement rates. By testing new subject lines and timing, it increases open rates by 20%.
4. Challenges in Data Analytics and How to Overcome Them
a. Data Quality Issues
Incomplete or inaccurate data can lead to flawed conclusions.
Solution: Implement robust data cleaning processes and use reliable data sources.
b. Data Overload
Too much data without clear focus can overwhelm decision-makers.
Solution: Define specific objectives and prioritize key performance indicators (KPIs).
c. Lack of Expertise
Not all businesses have in-house data analytics expertise.
Solution: Invest in training or partner with analytics consultants to bridge the skill gap.
d. Integration Across Systems
Fragmented data systems can hinder comprehensive analysis.
Solution: Use integration tools to consolidate data into a centralized platform for seamless analysis.
5. Real-World Applications of Data Analytics
a. Marketing Optimization
Businesses use analytics to measure campaign effectiveness and target the right audiences. For example:
- Social media platforms analyze user behavior to deliver targeted ads.
- Retailers use purchase history to recommend products.
b. Supply Chain Efficiency
Data analytics identifies inefficiencies in supply chain operations, helping reduce costs and improve delivery times.
Example: Amazon uses predictive analytics to optimize inventory levels and ensure timely deliveries.
c. Financial Forecasting
Financial institutions analyze historical data to predict market trends and assess investment risks.
d. Healthcare Insights
Healthcare providers use analytics to improve patient care and optimize resource allocation. For instance, predictive analytics helps identify patients at high risk of certain conditions.
6. Future Trends in Data Analytics
a. AI and Machine Learning Integration
AI-powered analytics tools can identify patterns and trends at unprecedented speeds, enabling real-time decision-making.
b. Big Data Expansion
As data volumes grow, businesses will increasingly adopt big data analytics to process and analyze massive datasets.
c. Enhanced Data Visualization
Advanced visualization tools will make it easier for decision-makers to interpret complex data.
d. Predictive and Prescriptive Analytics Growth
The shift toward predictive and prescriptive analytics will empower businesses to anticipate future trends and make proactive decisions.
Conclusion: Leveraging Data Analytics for Business Success
Data analytics is more than just a tool—it’s a strategy for transforming raw information into actionable insights that drive growth, efficiency, and innovation. By understanding the types of analytics, addressing challenges, and implementing best practices, businesses can make smarter, more informed decisions.
Whether you’re optimizing operations, improving customer experiences, or mitigating risks, data analytics equips you with the knowledge and confidence to navigate today’s competitive landscape. Start leveraging analytics today and unlock the full potential of your business.